Humans rely on eye sight and the processing of the resulting information in more everyday tasks than we realize. We are able to solve moderately difficult quadratic formulas in our head when taking information about a flying ball and aiming to hit it with a baseball bet at incredible speeds. We are able to use visual information about dozens of cars to navigate when driving a car in unknown streets. Our eyes calibrate to the lighting conditions allowing us to navigate broad daylight just as well as dimly lit rooms. Beyond that, we can use information about depth of field, color, tint, and sharpness. In fact, it is often said that over 50% of the cortex, the surface of the brain, is involved in vision processing tasks. This makes vision one of the most relied upon sense. Consequently, understanding what drives our eye movements may be a key to understanding how the brain as a whole works.
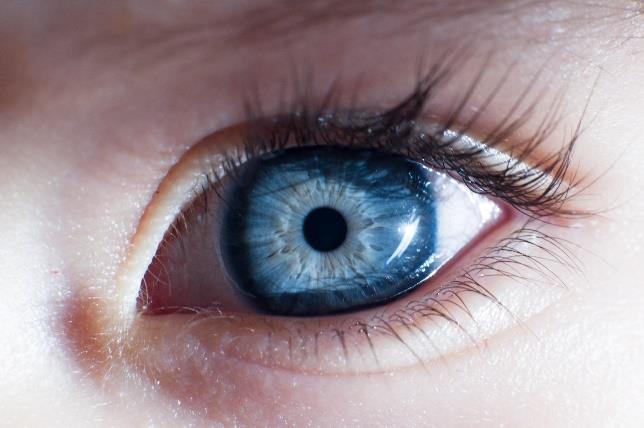
The eye’s movements and its anatomy
Eye movements are categorized in over ten different types of motion, two of which are most commonly studied: fixations and saccades. If the eye rests on a specific location for a certain amount of time, we classify this non-movement as a fixation. A fixation commonly lasts around 200 to 300 milliseconds during which the brain processes visual information. This information can be obtained from the entire visual field of about 200°, although the level of detail of information in 2° around the center is significantly higher. The movement from one fixation to the next is called a saccade and is the fastest eye movement – in fact the fastest movement the body can produce – with a duration between 30 to 80 ms and a speed of 30-500°/s (Holmqvist, et al., 2012). During the process of performing a saccade, no visual information is sent to the brain and, thus, it is safe to say that humans are effectively blind for duration of saccades.
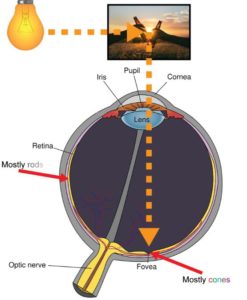
The reason that vision around the center of the field of view is of higher detail is the biological structure of the eye. There are two types of cells, called photoreceptors, that work together in resolving the light coming in through the lens into sensible information for the brain, called cones and rods. Cones are responsible for photopic vision meaning that they are active at higher light levels. They are also capable of color vision and resolve light information at higher levels of detail. The fovea, which is at the opposite side of the lens, is almost exclusively populated by these cones. Rods, on the other hand, handle scotopic vision meaning that they work at lower light levels. Rods do not mediate color vision and their spatial resolution capabilities are significantly smaller. Instead, rods are very sensitive to small variations in light (Hogan, Alvarado, & Weddell, 1971). Population of rods increases in areas further away from the fovea which is one of the reasons why, when stargazing, we can often detect dim stars in the night sky when we do not directly look at them. Figure 2: Light is reflected off of an object and enters the eye through the lens hitting some part of the eye. In the fovea a high population of cones means good resolution of colors and detail, while towards the sides a high population of rods ensures sensitivity to small changes of light. depicts the rough biological structure of the human eye.
Despite the fact that the central part of our vision has better spatial resolution, our brains are capable of shifting focus on parts of the image information coming through the optic nerve that is not in the central vision. Nonetheless, the location of the center of vision of a human observer is a good indicator for where his attention lies which is an idea that has been employed in human social interactions for thousands of years – following the gaze of another human being is a key concept in non-verbal language. It is a legitimate assumption that recording the movement of the eyes gives an insight into the brain’s attention steering mechanisms.
What we can learn from the position of the eyes?
In scientific work, however, human gaze has been studied since the 1800. Scientists would manually observe human eye movement and approximate the location of the gaze. Edmund Huey constructed an early and crude version of an eye tracker in the early 1900s in which specifically designed contact lenses with an attached aluminum pointer gave a better indicator of the orientation of the eye. In the 1950s Alred L. Yarbus worked on creating better, non-intrusive eye trackers that could give more accurate data on where people look. Despite the fact that portable video cameras were still in infancy, his groundbreaking research led to breakthroughs in understanding the relation of task dependency and eye movements as well as the impact of a subject’s interest on their fixation. Yarbus’ eye tracker from the 1960s is depicted in Figure 3: Alfred L. Yarbus’ eye tracker from the 1960s.. Since then, eye tracking has come a long way and is now performed using high-speed infrared cameras that record the eyes. Elaborate mathematical models then create a model of the eyes’ geometry and predict the location of the gaze in relation to the camera. With knowledge about the location of the camera, one can then accurately describe the predicted location on a computer screen, for example.
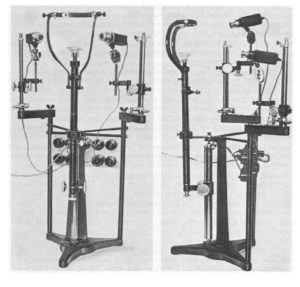
Through the quantification of various attributes of these eye movements we can make deductions about the behavior of the observer. The duration of a fixation, for instance, is a good indicator for cognitive activity during which the brain is processing visual information. It has been shown that fixation times for common words are significantly shorter than those of less common words (McConkie & Rayner, 1975). In another case it was shown that from gaze recordings of an observer of a video-recording of a driving task the participant’s skill in driving could be deduced directly (Underwood, Chapman, Bowden, & Crundall, 2002). Beyond this, eye tracking has been applied to great effects in numerous medical fields. Schizophrenia patients, for example, can be identified by an impairment in their ability to smooth pursuit (O’Driscoll & Callahan, 2008), an eye movement performed when tracking a plane in the sky. Similarly, low saccadic velocities occur frequently with Alzheimer’s disease and AIDS (Wong, 2008).
Beyond these cases, we have realized a lot about what parts of a scene are interesting to human observers, thanks to the technological advancements in our ability to tracking the eye. Human and animal faces, for example, are very noticeable taking up a vast majority of the time spent looking at an image. This is true even if they are partially or fully hidden (a great visualization of this can be seen here). Our brains are tuned to detect faces so much that we often see faces in inanimate objects, a phenomenon called pareidolia. As a consequence, numerous places around the world are famous for these sole resemblances, such as the Pedra da Gávea, an enormous rock outside Rio de Janeiro, Brazil, shown in Figure 4. Beyond faces, humans are keen to read text and inspect familiar or unfamiliar logos. In total, humans observe faces and text 16.6 and 11.1 times more frequently than other regions (Cerf, Frady, & Koch, 2009).
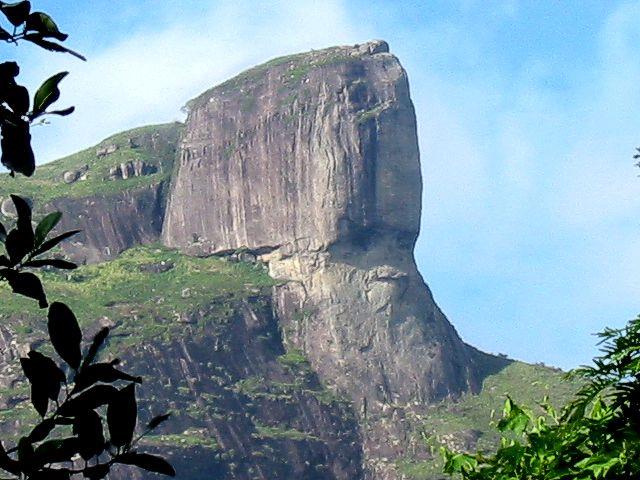
Commonly, when computer scientists have been trying to model the human visual system, they created distinct modules that had clear jobs. There was a module to recognize faces, one to recognize text, and so on. Recently, there has been a big movement in the scientific community to predic t eye movements made during image viewing tasks using complex computational models based on biology. The most sophisticated models attempt to replicate different layers of perception that are a reproduction of what is currently thought to be happening in the human brain itself. These deep convolutional neural networks (CNN) are organized according to the brain’s visual cortex using millions of binary units, much like neurons. What is especially cool about these CNNs is that different layers can be visualized meaning that we can get a glimpse into what appears to be happening in the different visual stimulus processing stages of our own brains. After training such a network on millions of images, scientists found that there are “neurons” in these artificial brains that activate for a variety of more or less complex tasks. They range from simple edges of certain directions to complex shapes resembling stars, faces, or eyes (Zeiler & Fergus, 2014). Investigating these models further will surely give deeper insight into what drives human visual processes.
Bibliography
Cerf, M., Frady, E. P., & Koch, C. (2009, November). Faces and text attract gaze independent of the task: Experimental data and computer model. Journal of Vision, 9(12).
Hogan, M. J., Alvarado, J. A., & Weddell, J. E. (1971). Histology of the Human Eye: an Atlas and Textbook. Philadelphia: W.B. Saunders Company.
Holmqvist, K., Nystrom, M., Andersson, R., Dewhurst, R., Jarodzka, H., & van der Weijer, J. (2012). Eye Tracking: A Comprehensive Guide to Methods and Measures. Oxford, UK: Oxford University Press.
McConkie, G. W., & Rayner, K. (1975, November). The span of the effective stimulus during a fixation in reading. Perception & Psychophysics, 17(6), pp. 578-586.
O’Driscoll, G. A., & Callahan, B. L. (2008, December). Smooth pursuit in schizophrenia: A meta-analytic review of research since 1993. Brain and Cognition, 68(3), pp. 359-370.
Underwood, G., Chapman, P., Bowden, K., & Crundall, D. (2002, June). Visual search while driving: skill and awareness during inspection of the scene. Transportation Research Part F: Traffic Psychology and Behaviour, 5(2), pp. 97-97.
Wong, A. M. (2008). Eye Movement Disorders. Oxford, UK: Oxford University Press.
Zeiler, M. D., & Fergus, R. (2014). Visualizing and Understanding Convolutional Networks. Computer Vision – ECCV 2014. Lecture Notes in Computer Science (pp. 818-833). Cham, CH: Springer.
Good Morning, I am a British trained Architect working for Northwestern University in Evanston Illinois. ( I also sent a short time at the Technische Universitat Darmstadt)
I am currently working on the design of a small laboratory for eye tracking research, for the study of Parkinson’s disease in elderly patients. The title of this article caught my attention. I was hoping to find data that would guide me on optimal environmental conditions for a lab of this nature. Very little is published on this topic. In particular I am looking at the acoustic separation of the lab from its surrounding environment., including what level of acoustic separation is optimal. Other areas of concern are: internal acoustic requirements/reverberation, HVAC noise, lighting levels/colour temperature, sun light penetration, temperature variations, colours, textures of finishes, etc., etc.
The majority of these items I can deal with as an Architect, however I am having some difficulty with the area of acoustic separation. My research in this subject is pointing to the connection between pupil and hearing channel size and how they are interconnected. In translating this into an architectural solution, and from experience, when you hear a sound, particularly a distracting sound you automatically turn your head to the direction of the sound. This very movement is something that will disrupt the user and hence the research, correct?
Given the location of this new laboratory, outside of a heavily used teaching lecture theatre, I have concerns about the degree of acoustic separation between the spaces. I would be interested to hear about your experiences regarding the necessary level of acoustic separation and any suggestions you have concerning this. The Professors I am working with are concerned about noise penetration into the room distracting the research subjects and their ability to concentrate. To date I have located very little on this subject, the assessments being on a subjective level rather that empirically driven. Have you or any of your subscribers any experience in this area? This might be an area that you cover in this blog to aid other researchers? It would be of great help to individuals such as I and other researchers
I would be very grateful if you could point me in the right direction. Any help you can give me is greatly appreciated.
Kindly.
Andrew